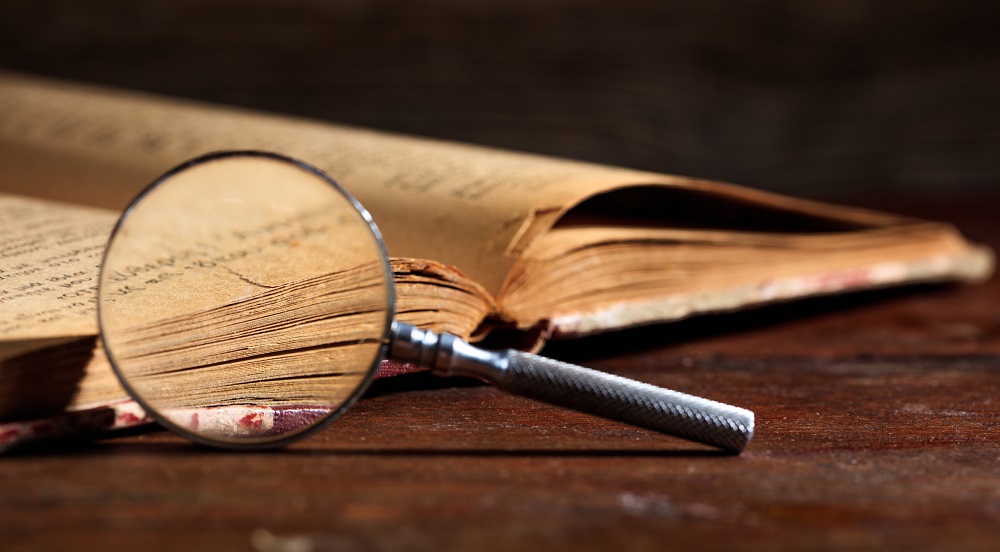
Tapping into Text Analytics
There’s no doubt that text analytics is a valuable tool in the insight arsenal. Well implemented, it can unlock meaning from unstructured data gathered from social media platforms, Voice of the Customer surveys, online reviews and many other sources.
The challenge for those looking to harness text analytics as part of a wider insight project is taking the time to understand what you want to achieve, with what kind of data, how much effort will be required, and the longer-term benefits to be derived from the investment.
We put a few text analytics tools under the microscope and found a marked difference in capabilities between tools available on the market. Here are our three key takeaways from our investigations:
- Look below the surface
Most demos of AI tools use data that they already know well. As a result, they appear easy to use, look efficient and seem to require only limited user input. However, not all AI verbatim coding tools are as good at analysing new data that they have not previously encountered which means there may be a requirement for much more time and effort to unlock the data than initially indicated.
- Weigh up the benefits
There is a clear trade-off between effort and return. Text analytics tools can of course be used for ‘one off’ data sets but the biggest benefit comes from using them for on-going projects where the set up and customisation involved makes it worthwhile. For some ad hoc projects, it can be quicker to stick to traditional methods of coding – unless the effort to develop the ‘ontology’ can be applied to multiple projects.
- It’s all about the data
The nature of the unstructured data you will be analysing will have a fundamental impact on the best tool for the job. Is it rich data or thin data? The volume of data is a factor; is there sufficient data to warrant the effort? Do you want to attach a score to verbatim comments, or other variables? Do the verbatims have any sentiment bias in them? If they do – such as “what do you like most/least” – bear in mind that sentiment analysis could be difficult.
Before you embark on the selection process, we recommend that you add these questions to your checklist:
- How much time and resources will be needed for customisation based on the uniqueness of the data, the terminology and the overlap to standard ontologies?
- Is the intention to create a more rapid coding tool, or do you really want to generate real insights from the data analysed?
- What level of sophistication do you need from the tool? How important is its capability to deliver INSIGHT and ACTION?
If you’d like to learn more about our assessment of text analytics tools and how we harness text analytics in insight projects for our clients, please get in touch.